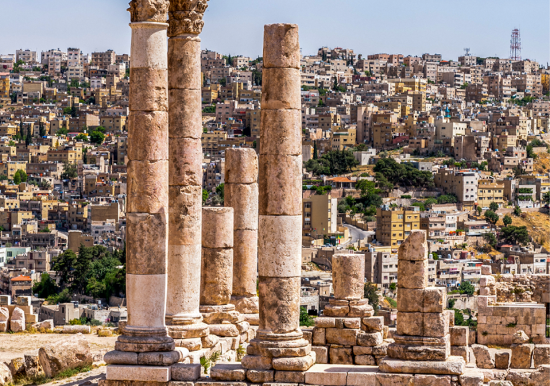
Connecting the Dots: Blended Innovation and the Art of Digitalisation in Governance
Reuben Ng, Assistant Professor, Lee Kuan Yew School of Public Policy and Lead Scientist at the Lloyd’s Register Foundation Institute for the Public Understanding of Risk
Dr. Reuben Ng spent 16 years in government, consulting, and research. In government, he was in the Prime Minister’s Office driving evidence-based policymaking through data analytics and Singapore’s Smart Nation strategies. In consulting, he co-built the advanced analytics practice at a top firm, and implemented complex analytics capabilities across industries and functions. In research, he specialises in quantitative social sciences and social gerontology, and has created innovative techniques to measure societal perceptions that are applied to strategic policy communications. Reuben delivers masterclasses on Innovation that blend Data Analytics, Behavioural Insights, and Design Thinking; he also serves on advisory boards in finance, education and sustainability.
Implementation Matters in Policymaking and Governance I am a psychologist by training, and I try to apply psychology and principles of human behaviour across different fields. I pursued my research interests in the US, working on projects regarding ageing and health. It was during the Obama administration, when the focus was to bring down healthcare costs while increasing healthcare outcomes. One of the ways to do this was through prevention—because prevention is typically cheaper than a cure.
Most of the time, prevention programmes do not work very well, because they tend to take a one-size-fits-all approach, even though people are very different. To address this, we worked with a concept called precision prevention or personalised prevention. This approach uses data to try to identify different behavioural segments in the community, to give us an idea of how to tweak programmes, their implementation, or communications to be as effective as possible to these different groups.
When I returned to Singapore, I transited to consulting with one of the big firms that was starting up its data and artificial intelligence (AI) practice. Most of our clients were governments in the Asia-Pacific. Later, I joined the Smart Nation Programme Office and Prime Minister’s Office’s Strategy Group before landing at the LKY School.
My experiences in these projects have taught me the importance of not being in a methodology silo: that we need to blend different approaches and data. I have come to realise that we sometimes try to get all the models right, but it is not just about the science of analytics or of AI. There is a craft to implementation that matters. We need to communicate, to convince senior management, to manage the change process. All these are part of the art of analytics as well, and vital to success.
Thinking Differently About Governance in the Digital Age
This goes to the skills we will need in ten, 20 years’ time. We think about making the workplace future ready, and about the regulation of new technologies. But relatively less work has been done on the skills we will need, especially in the public sector. In my view, there are a few common denominator skills governments could look at, to set civil servants up for the future.
The first is the notion of co-creation. Most of the time when we think about what people need, it is very policymaker centric. We think that is what other countries are doing, and we must keep up; or we think that is what citizens will need, so we create it for you. But we often forget to ask people what they need.
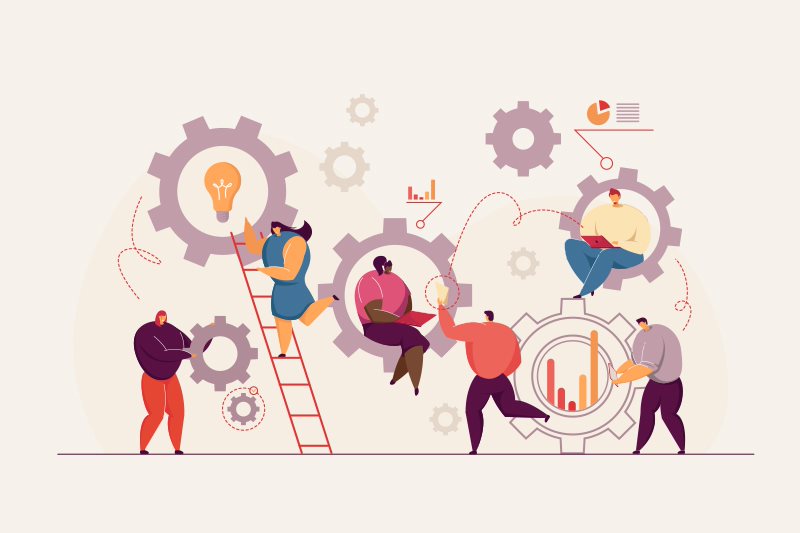
The same goes with international organisations that we have worked with, such as the World Bank. We have the resources and the plans, but it is critical to find out what the real needs are first. There was an example in a developing country where we were rolling out youth entrepreneurship programmes. They were not very successful, because the young people there just wanted to be good employees; not everyone wants to be an entrepreneur. Fundamentally, if we had understood and co-created with the local government, we could have used the money for many different pathways for the young people to be successful, rather than prescribing one track and putting all the funding into it.
So, there must be a thoughtful, skillful process of assessing needs. Co-creation needs to be the approach from the start—a part of this process, not an afterthought. This will be vital as governance goes digital.
Just because data is important does not mean everything can be solved with data. Instead, what I do is to take people through a problem case and see how approaches can be blended to solve it.
Another point is that the way governments make and implement policy tends to be quite siloed. There is not enough collaboration across the chain, and as a result we can get caught out and must react to things that happen which had in fact been anticipated by others long ahead of time. A fundamental question for the future is: how to build these bridges across different parts of the value chain? One way forward is to set up a policy sandbox, to try out some ideas and potential policy responses, so we can be more proactive than reactive. This will be important in managing new technologies.
It will also be important to take a blended innovation approach. Usually when we build capacity in government, it is about the flavour of the year. A couple of years ago it was design thinking, and now it might be behavioural insights. Next could be programme evaluation.
Blended Innovation: Using the Whole Toolkit to Solve Real Problems
A blended innovation approach simply means that we have an innovation toolkit that incorporates all the different tools we have acquired over the course of our careers, including the tools we have now. We do not just send people for “data” classes; we need to tell people what the data class is for, and how it connects with the earlier ideas and approaches we sent them to class for. This integrated perspective is often lacking. If you have a hammer, everything becomes a nail. Just because data is important does not mean everything can be solved with data. Instead, what I do is to take people through a problem case and see how approaches can be blended to solve it.
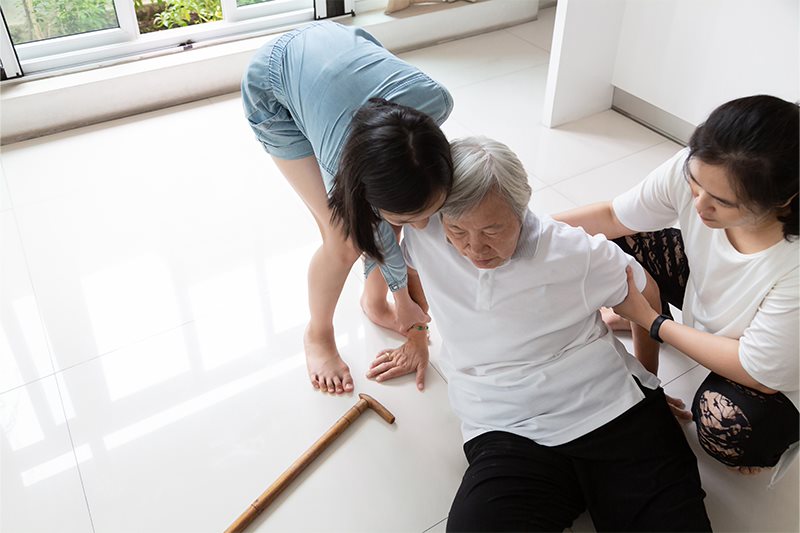
Here is an example. One of the bigger issues in healthcare in Singapore is seniors falling. Singapore has one of the highest community fall rates in the world; the incidence rate is about one in four. Some 80% of A&E visits by people aged 65 years and above is because of a serious fall. So, we want to address this and decrease the risk of people falling.
If we take a pure design thinking approach, we might choose two or three older adults, and follow them through the day to find out what the risk factors are for falls, and then think about how we might address them. Using a blended innovation approach I start instead with data. I use behavioural segmentation along with data analytics to understand how many types of older adults have fallen. Some of these cases might be because of the effect of medication, or because of the built environment. If there are three behavioural segments, for example, or three unique groups of older adults who may be susceptible to falls, then I will pick one or two in each segment and then do a design thinking patient journey with them. This gives me a better understanding of what the greatest potential risk factors are. Then I look for these risk factors in the data set, and I put them into my model.
The design thinking approach helps me come up with hypotheses of what to test in the data. The data tells us what the actual risks are, and which are modifiable. Then from there, I use behavioural insights to change what is modifiable. This is an example of how I have blended data, design thinking, and behavioural approaches. This blending is critical to producing an optimal solution. But these approaches are not often taught this way.
Connecting the Dots: From Knowledge to Application
Apart from the natural silos between departments, agencies, and disciplines, when we go for training, we do not often take an application-based approach. As a learner, I must join the dots myself, and sometimes I am at a loss: it is what I call the Friday–Monday chasm. You go for your courses on Friday, and you are done. You are psyched up and then on Monday you go back to work, and you realise you cannot apply what you have learnt. That is poor return on investment (ROI) for the money spent on training.
To bridge this Friday–Monday chasm, I have started what we call a policy innovation or data innovation lab, where the training focuses on not only equipping learners with skills, but also getting them to come up with proposals on how they can solve a particular problem or achieve an aspiration or stretch goal in their work, using the skills learned. Then they work for two to three months to produce a prototype or policy brief.
In the age of data, sometimes what is important is connecting the dots and telling the story. For instance, many good open data sets are out there, but people perceive them not to be open. One reason is that the story of the data and its significance has not been told, and so others form their own narratives based on their own impressions. For this reason, I have been encouraging our policymakers to tell our own Singaporean story, with data. If we do not do that, then there’ll be people outside of Singapore telling our story, probably without data, and we may not like it.
To be able to tell a meaningful story, using data, is part of what I call the art of digitalisation. The rest— the hardware and technical side of digitalisation—are hygiene factors.
Getting Good at the Art of Digitalisation
The art of digitalisation involves capabilities to do with co-creation, and with contextual needs assessment. We need design thinking to help us look at things from a citizen-centric perspective across processes and touch points. Behavioural insights are important, to help us understand and frame the messaging to increase uptake. These capabilities are taught—but often not in programmes to do with digitalisation. Take cybersecurity: very often, systems are less robust because of human behaviour. So, there is a need to think about how to get people to practise better cybersecurity behaviours, which could make use of these other approaches.
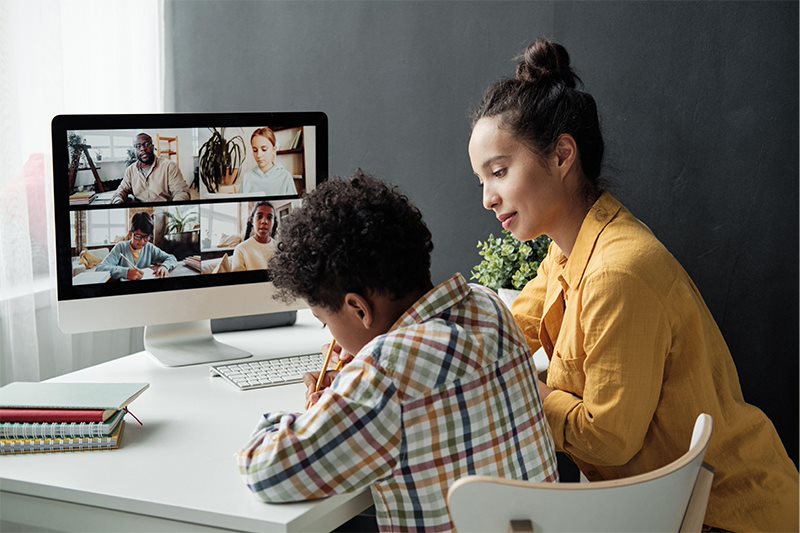
In the age of data, sometimes what is important is connecting the dots and telling the story
Dr. Reuben Ng, Assistant Professor, Lee Kuan Yew School of Public Policy
More Stories
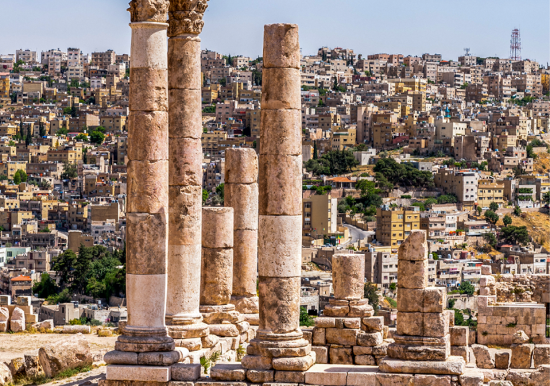
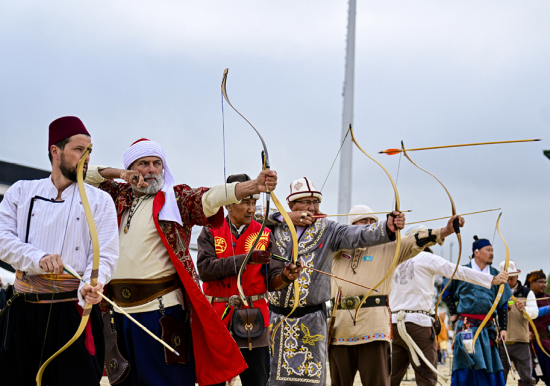
Global Influence & Reputation Country Snapshot: Türkiye
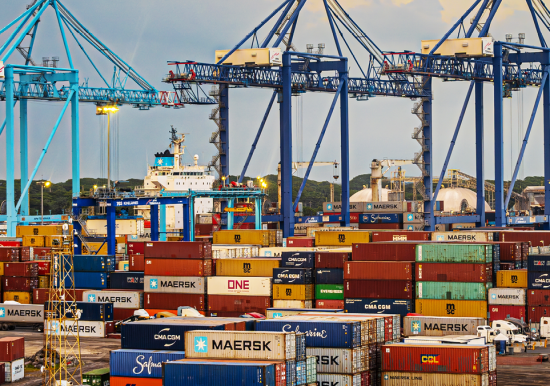